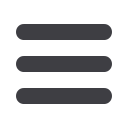
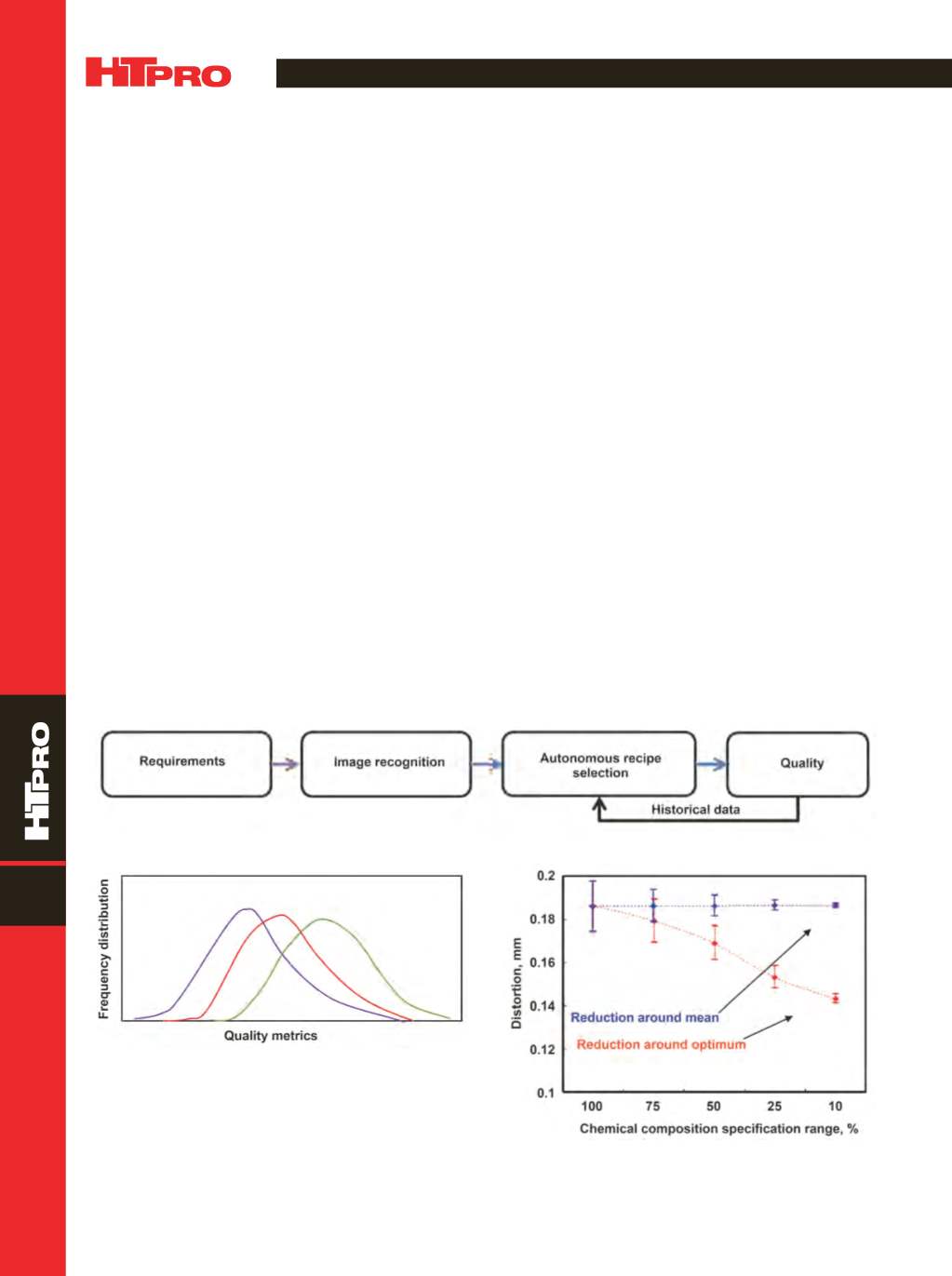
A D V A N C E D M A T E R I A L S & P R O C E S S E S | N O V E M B E R / D E C E M B E R 2 0 1 6
6 0
FEATURE
10
operating condition. Aligned with the overall Industry 4.0
vision, these changes would also make heat treating more
operationally efficient with robust, quality products.
INDUSTRY4.0: HEATTREATINGSTORYBOARDS
Three examples of Industry 4.0 will now be illustrated
for heat treating operations, described in an IoT framework
with key characteristics of sensing, connectivity, analytics
and intelligence capabilities, and business value:
Recipe management:
Although significant changes in
furnace technology and automation have occurred, the heat
treating recipe—which is the core of heat treating opera-
tions—continues tobeheuristicallydeveloped. Furthermore,
recipe selection for new parts is also heuristic, resulting in a
significant amount of rework and reject levels at initial stages
of production. It is expected that Industry 4.0would have the
most significant impact on how heat treating recipes are
developed and managed. Recipe selection would primarily
be accomplished through image recognition algorithms of a
part, which would eliminate mistakes in selection. Further,
self-learning algorithms would enable continuous evolu-
tion of recipes with feedback from historical quality data
(Fig. 4). This would also enable recipe customization based
on the current part chemistry and furnace health and oper-
ating condition. The system would also have self-evolving
capabilities for heat treating recipes to become rationalized
(Fig. 5) over time due to changes in product mix. Any recipe
decision due to production disruption in pre- or post-steps
could be better managed. Consequently, heat treating oper-
ations will be more efficient and product quality will be pre-
dictable. In the Industry 4.0 framework, heat treaters need:
sensing capability for part shape, quantity, and weight in the
furnace; connectivity and analytics capability to choose the
correct reference recipe; intelligence capability to fine-tune
the recipe based on instantaneous inputs and operating
conditions; and long-term rationalization and recipe devel-
opment to create value in terms of efficient operations and
robust product quality.
Distortion control:
Distortion control in precision trans-
mission components, such as gears and shafts, is a signifi-
cant heat treating challenge. Critical parameters including
chemistry (Fig. 6), process parameters during reheating
and forging, annealing and machining conditions, and car-
burizing and quenching conditions impact distortion. A key
reason for distortion is inadequate information at different
processing steps, which prevents efficient distortion-man-
agement strategies. For example, chemical composition is
directly linked to hardenability and distortion. Significant
control of distortion could be achieved if information on
the composition is known at the heat treating step, and an
instantaneous recipe created incorporating this information.
Further, depending on quench-tank conditions and furnace
Fig. 4 —
Self-learning algorithms and intelligence created for autonomous recipe selection, management, rationalization, and fine-tuning.
Fig. 5 —
Overlapping frequency distributions of quality metrics
obtained fromdifferent recipes indicate the possibility of recipe
rationalization.
Fig. 6 —
Influence of steel chemical composition (within specified
chemistry range) on heat treating distortion, which presents an
opportunity to control distortion by fine-tuning recipes for specific
loads.