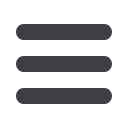
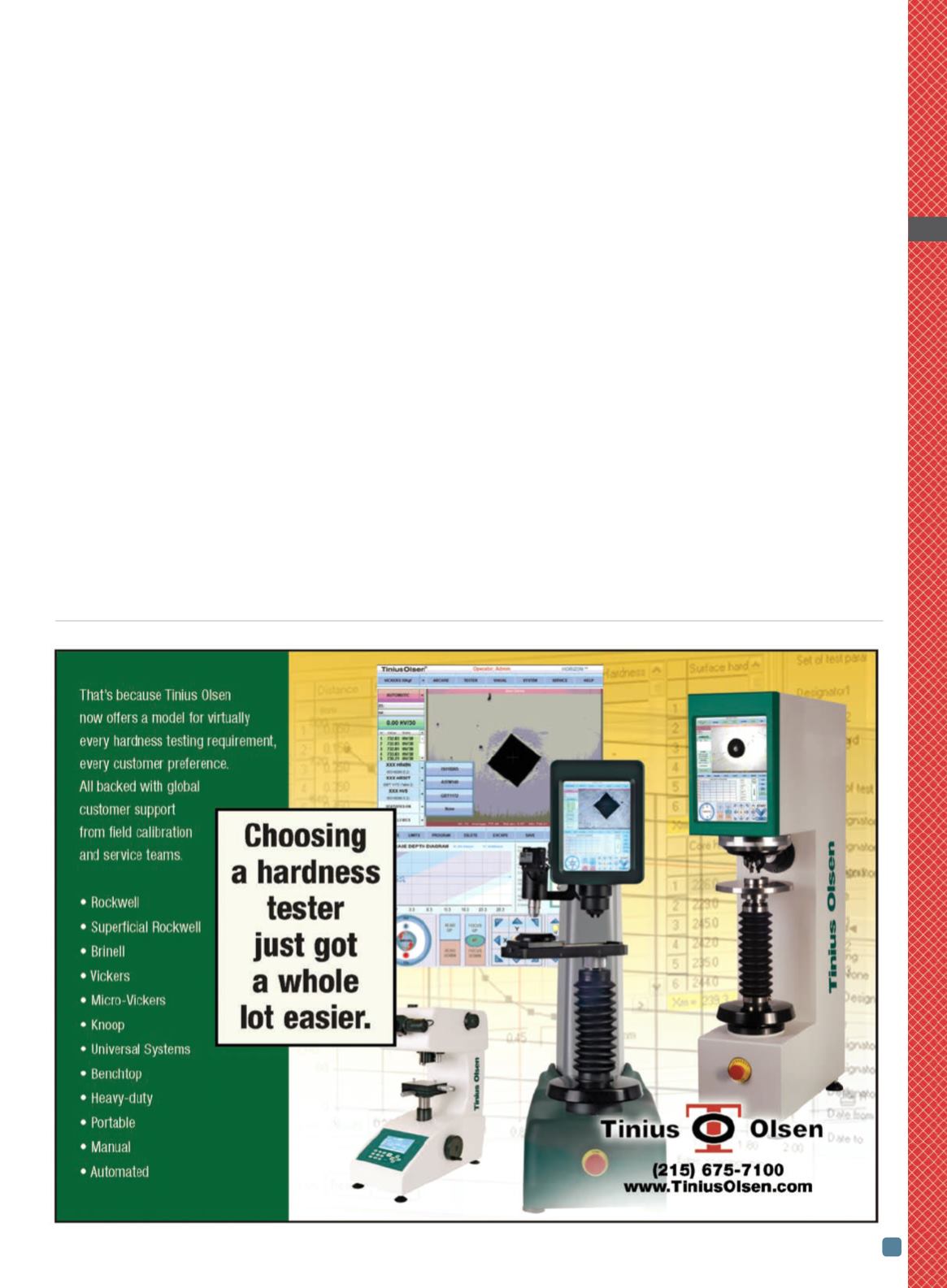
ADVANCED MATERIALS & PROCESSES •
MARCH 2014
47
fusion kinetics and neural network
modeling.
Major deployable outcomes included
a new operating regime, new recipes,
and a recipe selection methodology.
The study projected a 12.5% produc-
tivity increase, together with ease of
operation (lower number of recipes),
specific energy consumption, and 90
MT per year of carbon footprint re-
duction, which is equivalent to 15 au-
tomobiles with an average of 12,000
miles/year at 26 mpg fuel efficiency.
These examples demonstrate the ef-
fectiveness of the approach and the
value realized at the shop floor, which
justify the efforts needed for execut-
ing such projects. Better use of this
methodology requires standardiza-
tion of data, models, and an analysis
approach. Although most modern
processes have transitioned to IT in-
frastructures where data is collected,
data consolidation and standardiza-
tion remains an issue. Modeling ap-
proaches have not matured into
standard software products, such as
in-design, finite element analysis
(FEA), and computational fluid dy-
namics (CFD). Selecting the right ap-
proach, developing models, and
analyzing results to generate deploy-
able solutions requires a significant
level of technical expertise with an
understanding of first principles and
mathematical modeling. The greatest
limitation in this area is finding the
technical talents with the necessary
level of expertise.
Conclusion
A significant amount of data is gener-
ated from modern manufacturing oper-
ations, which can be effectively
leveraged together with heat treating
first principles understanding for ana-
lytics, modeling, and optimization of
heat treating operations aimed at re-
ducing specific energy consumption
and improving productivity and prod-
uct quality for an overall reduction in
operating costs. The approach is proven
with value realized on the shop floor,
but it has not completely matured for
standard deployment due to a lack of
necessary technical expertise.
HTPRO
References
1. J. Davis, et al., Smart manufacturing,
manufacturing intelligence and demand
dynamic performance, Foundations of
Computer-Aided Process Operations 2012
Conf., Savannah, Ga., p 1-18, 2012.
2. S.S. Sahay, et al., Process Analytics,
Modeling, and Optimization of an Indus-
trial Batch Annealing Operation,
Materi-
als and Manufacturing Processes
, Vol 24,
p 1459-1466, 2009.
3. S.S. Sahay, et al., Model-based optimisa-
tion of a highly automated industrial batch
annealing operation,
Ironmaking Steelmak-
ing
, Vol 33, No. 4, p 306-314, 2006.
For more information:
Satyam S. Sahay,
John Deere Asia Technology Innovation
Center, Cybercity, Magarpatta City, Pune
411013 India,
satyamsahay@yahoo.com.
Robert Gaster, John Deere Moline Tech-
nology Innovation Center, One John Deere
Place, Moline, IL 61265, USA, 309/765-
3741,
gasterrobertj@johndeere.com,
www. johndeere.com.
HTPRO
11