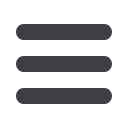
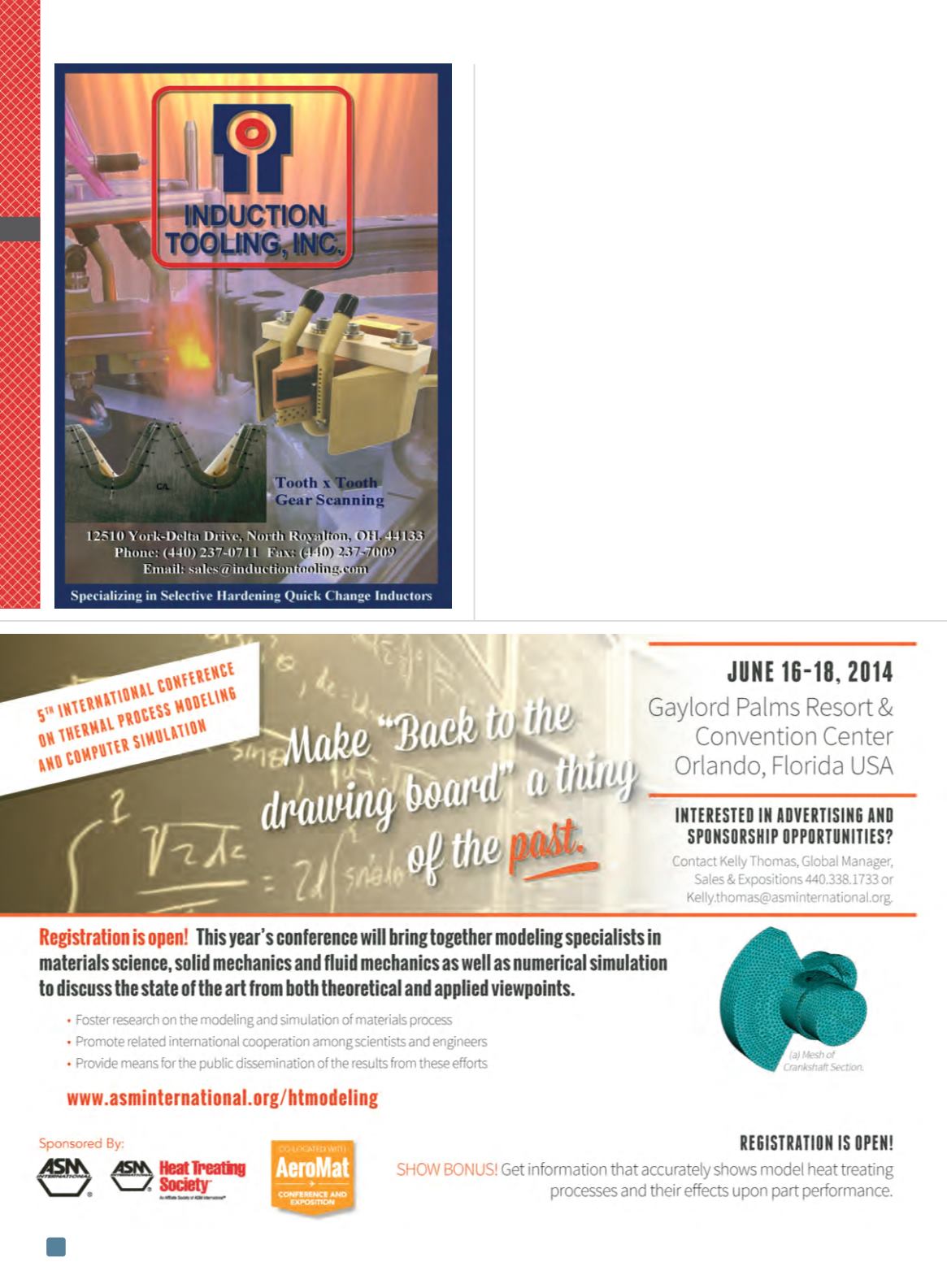
ADVANCED MATERIALS & PROCESSES •
MARCH 2014
46
and quality data for analysis. The reconciled and merged
data were used for further analyses.
Some key analyses included:
• Checking inputs and quality against specifications
• Checking the consistency of various recipes
• Principal component analysis (PCA) to find key input
variables that impact quality parameters
• Using neural networks to relate input variables with
quality
• Using first principle models (including diffusion kinetics)
for consistent validation and selection of recipes
The operation had very high emphasis on product quality,
with every normal batch meeting the specified product
quality. However, minor process parameter variations
were observed, which were correlated to case-depth vari-
ation (within the quality specification). Results of detailed
data analysis and the diffusion model indicated that
recipes with overlapping specifications could be rational-
ized to reduce the number of required recipes. Further-
more, principal component analysis helped in segregating
process parameters having less influence on quality data.
This was used to build a robust mathematical model. An
artificial neural network model was built based on key pa-
rameters, which could predict 98% of the case depth for
any combinations of process parameters within an uncer-
tainty of 20%, which was consistent with the plant data.
In addition, process parameters were optimized using dif-
HTPRO
10