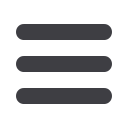
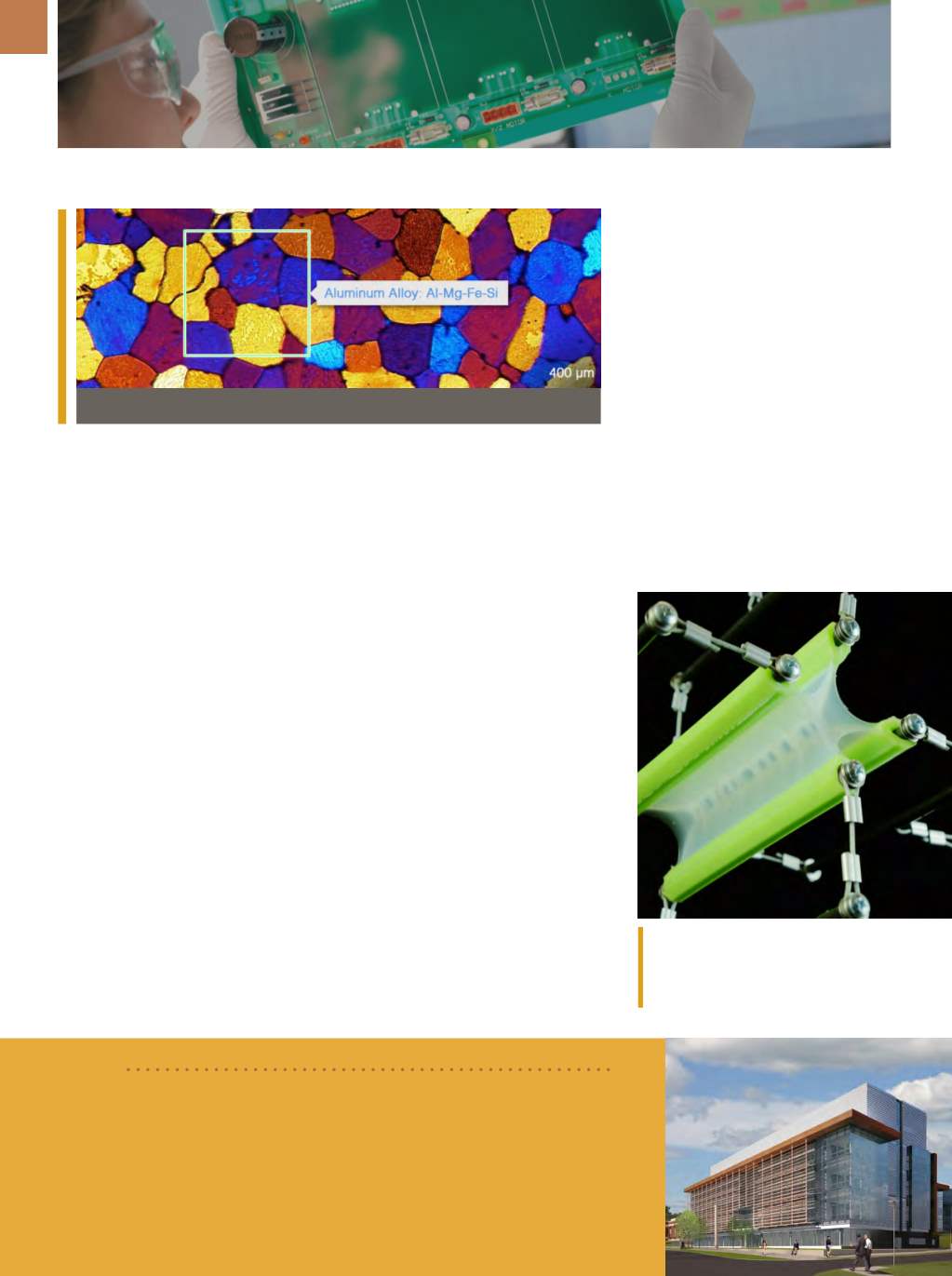
A D V A N C E D M A T E R I A L S & P R O C E S S E S | N O V E M B E R / D E C E M B E R 2 0 1 6
1 2
MACHINE LEARNING
LEARNS MATERIALS
In a field that has historically relied
on human experts to identify research
images by hand, a materials science
professor at Carnegie Mellon Univer-
sity, Pittsburgh, developed a system
that uses machine learning algorithms
to search, sort, classify, and identify
visual data sets in materials science.
Professor Elizabeth Holm is using the
algorithms—familiar to Google image
search users—to automatically recog-
nize and categorize images of micro-
structures. Because the algorithms are
designed to improve as they encounter
more data, they are especially suited
to the vast image libraries amassed in
materials science.
Holm’s machine learning system
could find application in research, indus-
try, publishing, and academia. For exam-
ple, it could be used to search scientific
journal archives to determine if a photo
was previously published, or automat-
ically categorize visual data for indus-
tries or research labs. “Big companies
can have archives of more than 600,000
research images,” explains Holm. “No
one wants to look through those, but
they want to use that data to better un-
derstand their products.” The next step
is application in metal 3D printing. Holm
and her team are compiling a database
of experimental and simulated metal
powder micrographs in order to better
understand what types of raw materials
are most appropriate for additive manu-
facturing processes.
cmu.edu.
INSTABILITY PREDICTION
PROVEN AFTER LONG STRETCH
Researchers at the Harvard John
A. Paulson School of Engineering and
Applied Sciences (SEAS), Cambridge,
Mass., demonstrated for the first time
that a decades-old theory proves true:
With the right amount of tensile force, a
thick cube of soft material will sudden-
ly deform into a thin, flat plate. In 1948,
applied mathematician Ronald Rivlin
predicted the behavior, but materials
scientists had been unable to prove the
theory experimentally—until now. The
Google-like algorithms are now being used to categorize micrographs.
Under biaxial tension, the elastomer
sample’s center deforms, breaking the
symmetry and becoming flat. Courtesy
of Harvard SEAS.
BRIEF
Construction is underway on the new Materials Design Laboratory (MDL) at the DOE’s
Argonne National Laboratory,
Lemont, Ill. The facility will complete Argonne’s
Energy Quad, a group of four adjoining buildings designed to maximize collaboration
between energy and materials scientists. Encompassing roughly 115,000 sq. ft. of
laboratory and office space, including 10,000 sq. ft. of lab space for radiological
research, the MDL is on track to receive LEED Gold certification. The lab will house
microstructure research and materials testing under extreme conditions.
anl.gov.
The newMaterials Design Laboratory at Argonne National Laboratory broke ground in September.
Harvard team triggered this instability
in a centimeters-thick elastomer block,
deforming it into a flat surface. To do so,
they stretched a thick, soft elastomer
made of silicon rubber at each corner.
Under biaxial tension, the sample’s cen-
ter deformed, breaking the geometrical
symmetry and becoming suddenly flat.
“This research widens the design
space for newarchitectedmaterials that
use instabilities to change or enhance
their functionality,” says Johannes T.B.
Overvelde, former graduate student at
SEAS. “With this instability, we can cre-
ate materials that can suddenly switch
between behaviors by using simple
triggers to change their geometry.”
harvard.edu.
EMERGING TECHNOLOGY